Artificial intelligence in endodontics: relevant trends and practical perspectives
DOI:
https://doi.org/10.56569/UDJ.2.1.2023.96-101Keywords:
artificial intelligence, endodontics, review, image processing, computer-assistedAbstract
Background. Overall pool of studies regarding artificial intelligence (AI) implementation in dentistry is increasing every year, while possibilities for using AI methods within everyday endodontic practice is still quite confined and not always enough affirmed.
Objective. To systematize and depict principal data regarding use of virtual artificial intelligence for various endodontic-related clinical purposes.
Materials and Methods. Targeted literature search was provided within National Center for Biotechnology Information databases using pre-specified Mesh-terms algorithm. The following information was extracted from each publication during content analysis: diagnostic and treatment planning aspects of endodontic practice for which AI methods could be applied; accuracy levels registered for AI models used for different endodontic-related purposes; limitations of using AI within endodontic practice.
Results. AI features could be used in endodontic practice for the following reasons: analysis of root canal morphology, identification of root fractures, verification of periapical lesions, estimation of root canal working length, root canal treatment planning, prediction of pain development during post-treatment period, predication of endodontic interventions success. The most prevalently used artificial intelligence methods for different endodontic diagnostic and treatment planning objectives were the following: convolutional neural network, artificial neurons network, case-based reasoning, deep learning, machine learning, neuro-fuzzy inference system, probabilistic neural network.
Conclusion. Main advantage of using AI models in endodontic practice associated with improvement of diagnostic accuracy within reduced amount of time needed for X-ray images and clinical data analysis. AI application for apical foramen detection and working length determination demonstrates the highest level of accuracy compared to AI performance for other clinically related objectives in endodontics.
References
Khanagar SB, Alfadley A, Alfouzan K, Awawdeh M, Alaqla A, Jamleh A. Developments and Performance of Artificial Intelligence Models Designed for Application in Endodontics: A Systematic Review. Diagnostics. 2023;13(3):414. doi: https://doi.org/10.3390/diagnostics13030414
Karobari MI, Adil AH, Basheer SN, Murugesan S, Savadamoorthi KS, Mustafa M, Abdulwahed A, Almokhatieb AA. Evaluation of the Diagnostic and Prognostic Accuracy of Artificial Intelligence in Endodontic Dentistry: A Comprehensive Review of Literature. Comput Math Methods Med. 2023;2023:7049360. doi: https://doi.org/10.1155/2023/7049360
Schwendicke FA, Samek W, Krois J. Artificial intelligence in dentistry: chances and challenges. J Dent Res. 2020;99(7):769-74. doi: https://doi.org/10.1177/0022034520915714
Rodrigues JA, Krois J, Schwendicke F. Demystifying artificial intelligence and deep learning in dentistry. Braz Oral Res. 2021;35:e094. doi: https://doi.org/10.1590/1807-3107bor-2021.vol35.0094
Sadr S, Mohammad-Rahimi H, Motamedian SR, Zahedrozegar S, Motie P, Vinayahalingam S, Dianat O, Nosrat A. Deep learning for detection of periapical radiolucent lesions: a systematic review and meta-analysis of diagnostic test accuracy. J Endod. 2023;49(3):248-61. doi: https://doi.org/10.1016/j.joen.2022.12.007
Hiraiwa T, Ariji Y, Fukuda M, Kise Y, Nakata K, Katsumata A, Fujita H, Ariji E. A deep-learning artificial intelligence system for assessment of root morphology of the mandibular first molar on panoramic radiography. Dentomaxillofac Radiol. 2019;48(3):20180218. doi: https://doi.org/10.1259/dmfr.20180218
Aminoshariae A, Kulild J, Nagendrababu V. Artificial intelligence in endodontics: current applications and future directions. J Endod. 2021;47(9):1352-7. doi: https://doi.org/10.1016/j.joen.2021.06.003
Boreak N. Effectiveness of artificial intelligence applications designed for endodontic diagnosis, decision-making, and prediction of prognosis: a systematic review. J Contemp Dent Pract. 2020;21(8):926-34.
Fatehi F, Gray LC, Wootton R. How to improve your PubMed/MEDLINE searches: 3. advanced searching, MeSH and My NCBI. J Telemed Telecare. 2014;20(2):102-12. doi: https://doi.org/10.1177/1357633X13519036
Kim S, Yeganova L, Wilbur WJ. Meshable: searching PubMed abstracts by utilizing MeSH and MeSH-derived topical terms. Bioinformatics. 2016;32(19):3044-6. doi: https://doi.org/10.1093/bioinformatics/btw331
Mao Y, Lu Z. MeSH Now: automatic MeSH indexing at PubMed scale via learning to rank. J Biomed Semantics. 2017;8(1):15. doi: https://doi.org/10.1186/s13326-017-0123-3
Umer F, Habib S. Critical analysis of artificial intelligence in endodontics: a scoping review. J Endod. 2022;48(2):152-60. doi: https://doi.org/10.1016/j.joen.2021.11.007
Hu Z, Cao D, Hu Y, Wang B, Zhang Y, Tang R, Zhuang J, Gao A, Chen Y, Lin Z. Diagnosis of in vivo vertical root fracture using deep learning on cone-beam CT images. BMC Oral Health. 2022;22(1):382. doi: https://doi.org/10.1186/s12903-022-02422-9
Johari M, Esmaeili F, Andalib A, Garjani S, Saberkari H. Detection of vertical root fractures in intact and endodontically treated premolar teeth by designing a probabilistic neural network: an ex vivo study. Dentomaxillofac Radiol. 2017;46(2):20160107. doi: https://doi.org/10.1259/dmfr.20160107
Hu Y, Cao D, Wang B, Chen Y, Lin Z. Preliminary Exploration of Artificial Intelligence in Diagnosis of Displaced Root Fracture Based on Convolutional Neural Network. J Oral Sci Res. 2022;38(10):959-62. doi: https://doi.org/10.13701/j.cnki.kqyxyj.2022.10.012
Fukuda M, Inamoto K, Shibata N, Ariji Y, Yanashita Y, Kutsuna S, Nakata K, Katsumata A, Fujita H, Ariji E. Evaluation of an artificial intelligence system for detecting vertical root fracture on panoramic radiography. Oral Radiol. 2020;36:337-43. doi: https://doi.org/10.1007/s11282-019-00409-x
Orhan K, Bayrakdar IS, Ezhov M, Kravtsov A, Özyürek TA. Evaluation of artificial intelligence for detecting periapical pathosis on cone‐beam computed tomography scans. Int Endod J. 2020;53(5):680-9. doi: https://doi.org/10.1111/iej.13265
Setzer FC, Shi KJ, Zhang Z, Yan H, Yoon H, Mupparapu M, Li J. Artificial intelligence for the computer-aided detection of periapical lesions in cone-beam computed tomographic images. J Endod. 2020;46(7):987-93. doi: https://doi.org/10.1016/j.joen.2020.03.025
Calazans MA, Ferreira FA, Alcoforado MD, Santos AD, Pontual AD, Madeiro F. Automatic Classification System for Periapical Lesions in Cone-Beam Computed Tomography. Sensors. 2022;22(17):6481. doi: https://doi.org/10.3390/s22176481
Albitar L, Zhao T, Huang C, Mahdian M. Artificial Intelligence (AI) for Detection and Localization of Unobturated Second Mesial Buccal (MB2) Canals in Cone-Beam Computed Tomography (CBCT). Diagnostics. 2022 Dec 18;12(12):3214. doi: https://doi.org/10.3390/diagnostics12123214
Buyuk C, Arican Alpay B, Er F. Detection of the separated root canal instrument on panoramic radiograph: a comparison of LSTM and CNN deep learning methods. Dentomaxillofac Radiol. 2023;52(3):20220209. doi: https://doi.org/10.1259/dmfr.20220209
Jeon SJ, Yun JP, Yeom HG, Shin WS, Lee JH, Jeong SH, Seo MS. Deep-learning for predicting C-shaped canals in mandibular second molars on panoramic radiographs. Dentomaxillofac Radiol. 2021;50(5):20200513. doi: https://doi.org/10.1259/dmfr.20200513
Yang S, Lee H, Jang B, Kim KD, Kim J, Kim H, Park W. Development and validation of a visually explainable deep learning model for classification of C-shaped canals of the mandibular second molars in periapical and panoramic dental radiographs. J Endod. 2022;48(7):914-21. doi: https://doi.org/10.1016/j.joen.2022.04.007
Gao X, Xin X, Li Z, Zhang W. Predicting postoperative pain following root canal treatment by using artificial neural network evaluation. Sci Rep. 2021;11(1):17243. doi: https://doi.org/10.1038/s41598-021-96777-8
Campo L, Aliaga IJ, De Paz JF, García AE, Bajo J, Villarubia G, Corchado JM. Retreatment predictions in odontology by means of CBR systems. Comput Intell Neurosci. 2016;2016:7485250. doi: https://doi.org/10.1155/2016/7485250
Bindal P, Bindal U, Kazemipoor M, Jha SK. Hybrid machine learning approaches in viability assessment of dental pulp stem cells treated with platelet-rich concentrates on different periods. App Med Inform. 2019;41(3):93-101.
Mohan SP, Ramalingam M. Dental pulp stem cells in neuroregeneration. J Pharm Bioallied Sci. 2020;12(Suppl 1):S60-S66. doi: https://doi.org/10.4103/jpbs.JPBS_229_20
Richert R, Ducret M, Alliot‐Licht B, Bekhouche M, Gobert S, Farges JC. A critical analysis of research methods and experimental models to study pulpitis. Int Endod J. 2022;55:14-36. doi: https://doi.org/10.1111/iej.13683
Ramezanzade S, Laurentiu T, Bakhshandah A, Ibragimov B, Kvist T, Bjørndal L. The efficiency of artificial intelligence methods for finding radiographic features in different endodontic treatments-a systematic review. Acta Odontol Scand. 2022;1-4. doi: https://doi.org/10.1080/00016357.2022.2158929
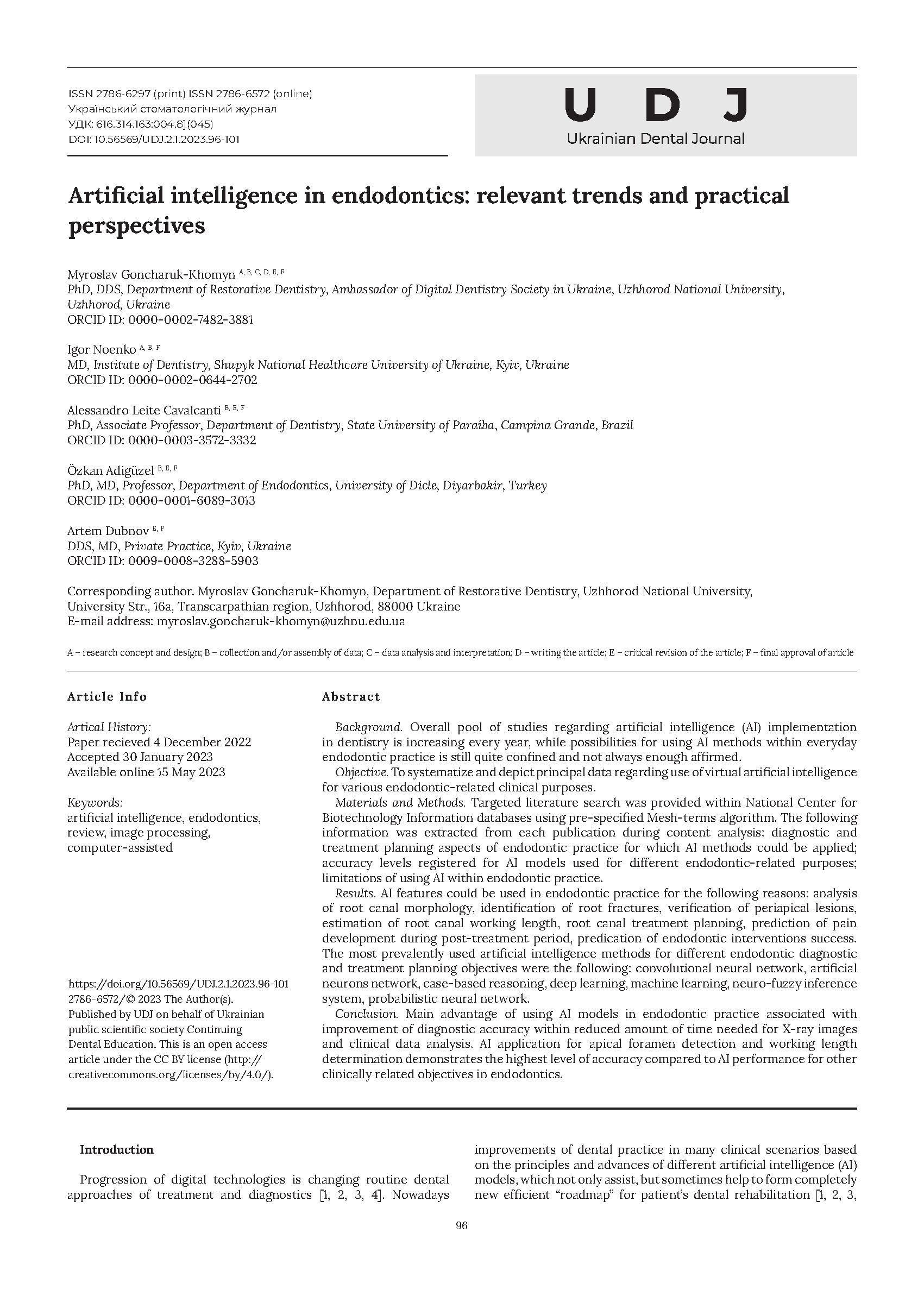
Downloads
Published
Issue
Section
Categories
License
Copyright (c) 2023 The Author(s)

This work is licensed under a Creative Commons Attribution 4.0 International License.